Passenger’s deep learning research with Bournemouth University
Our doctoral research work with Bournemouth University into the development of novel deep-learning methods for the prediction of arrival times in urban bus networks.
23rd Oct 2022
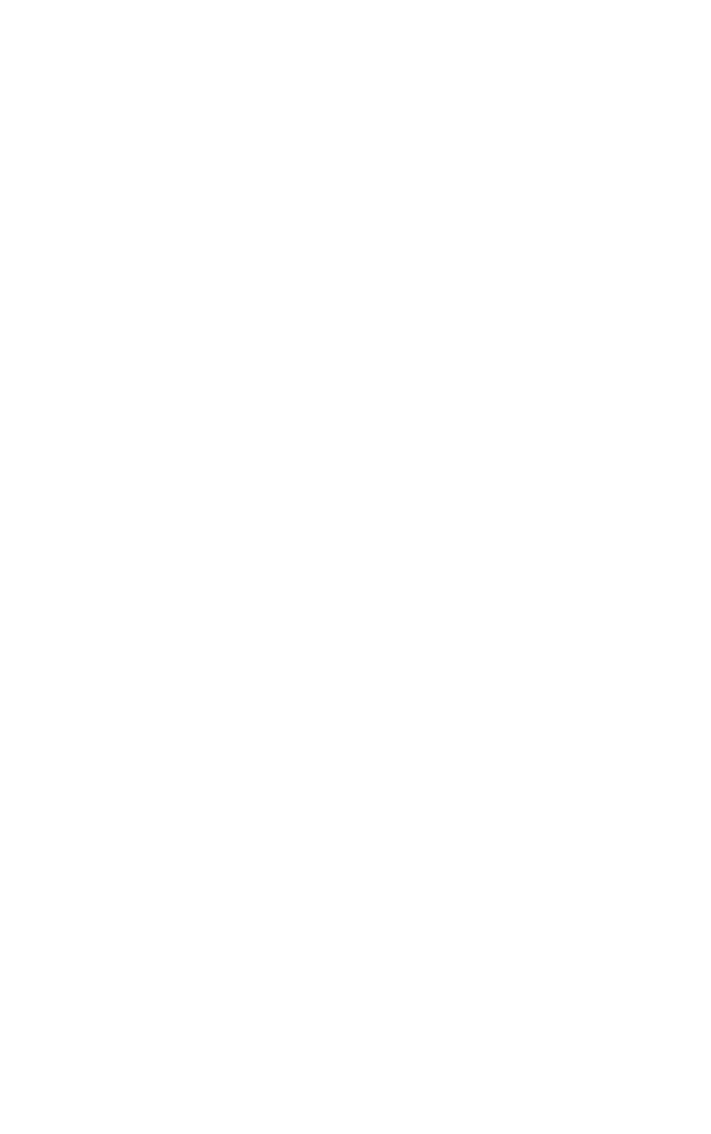
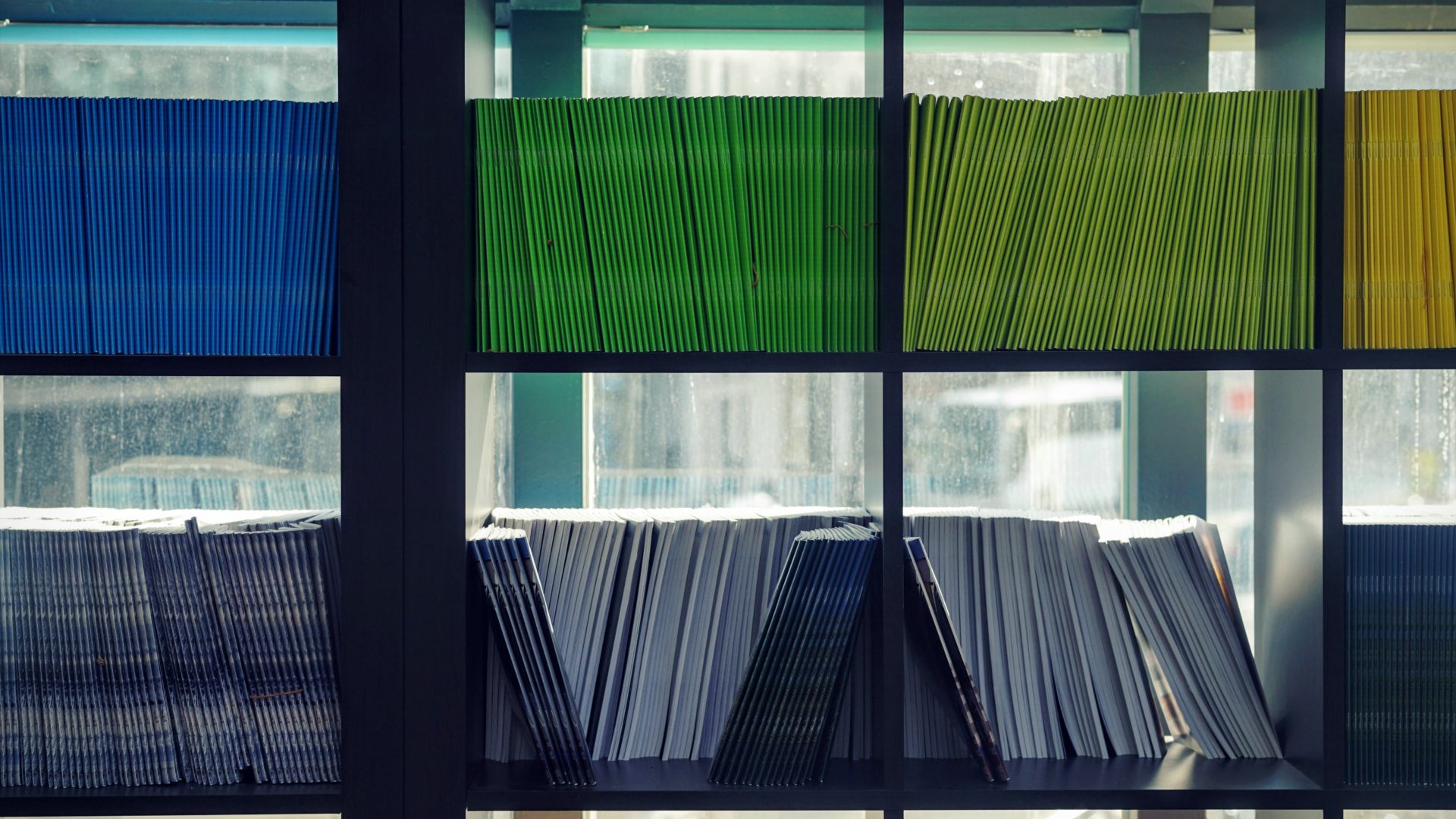
Providing accurate and useful journey information to people wanting to take shared transport is something we’re passionate about and is key to our success as the leading provider of transport apps.
Academic research is an important part of our strategy and we’re delighted to share that Bournemouth University’s Thilo Reich has now completed his doctoral research into the development of novel deep-learning methods for the prediction of arrival times in urban bus networks. Thilo’s transport-focused PhD, “Sequence modelling using deep learning approaches for spatial temporal public transport data” was supported by Passenger’s Principle Engineer Dave Hulbert, and Marcin Budka, Professor of Data Science at Bournemouth University.
Distilling the focus of his PhD, Thilo explains, “With public transport data, you have a bus that starts its journey. And at the beginning of the journey, you don’t know much about the bus. You know which line it’s on and what timetable it should adhere to. But you don’t know much else. Over time, you accumulate more data; where the bus has been, whether it has been at a bus stop, and so on, and that’s the sequence part of the title. A sequence is generated based on the journey that the vehicle is making.”
The PhD explored using machine learning methods, and artificial intelligence to improve arrival time predictions. The thinking was that including what happens in the entire city or the network, the spatial-temporal, could improve the prediction. If some sort of disruption occurred on line number two, for example, it would propagate through to eventually affect line number one; when something happens in one part of the network it creates a ripple effect. Because it’s all connected.
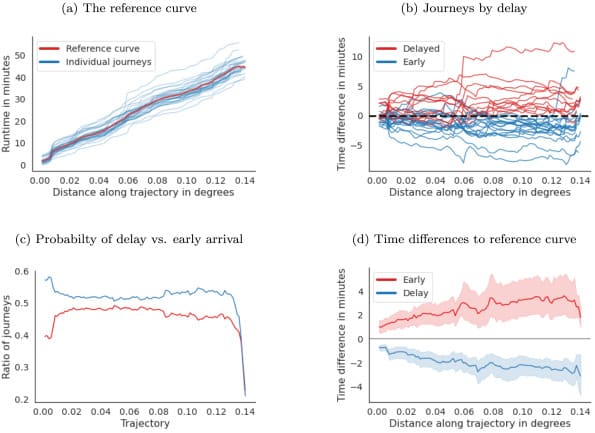
Reliable predictions of current vehicle position and arrival times play a crucial part in making public transport by bus as convenient as possible. However, this is being inhibited by the lack of reliable data, making any such algorithm development difficult. According to Marcin, one of the issues causing inconsistent information is undocumented quirks in proprietary systems, such as ticket machines behaving in a certain way when they think a bus is approaching a bus stop.
Earlier research, Bus journey simulation to develop public transport predictive algorithms, compiled by Marcin, Thilo and Dave, describes a method of generating realistic journeys that builds a bridge between low-quality recordable data and the real world. As a result, they have created a platform to develop algorithms in a simulated and controlled environment, which can later be deployed in a real-world scenario.
Passenger’s relationship with Bournemouth University’s data science faculty goes back to 2015 when the partner organisations embarked on a 2 year Knowledge Transfer Partnership (KTP) funded by Innovate UK, fusing academic research with commercial capability. The KTP was designed to develop and embed innovative data analytics and machine learning capabilities across the software development team at Passenger in order to enhance existing and create novel data-driven products.
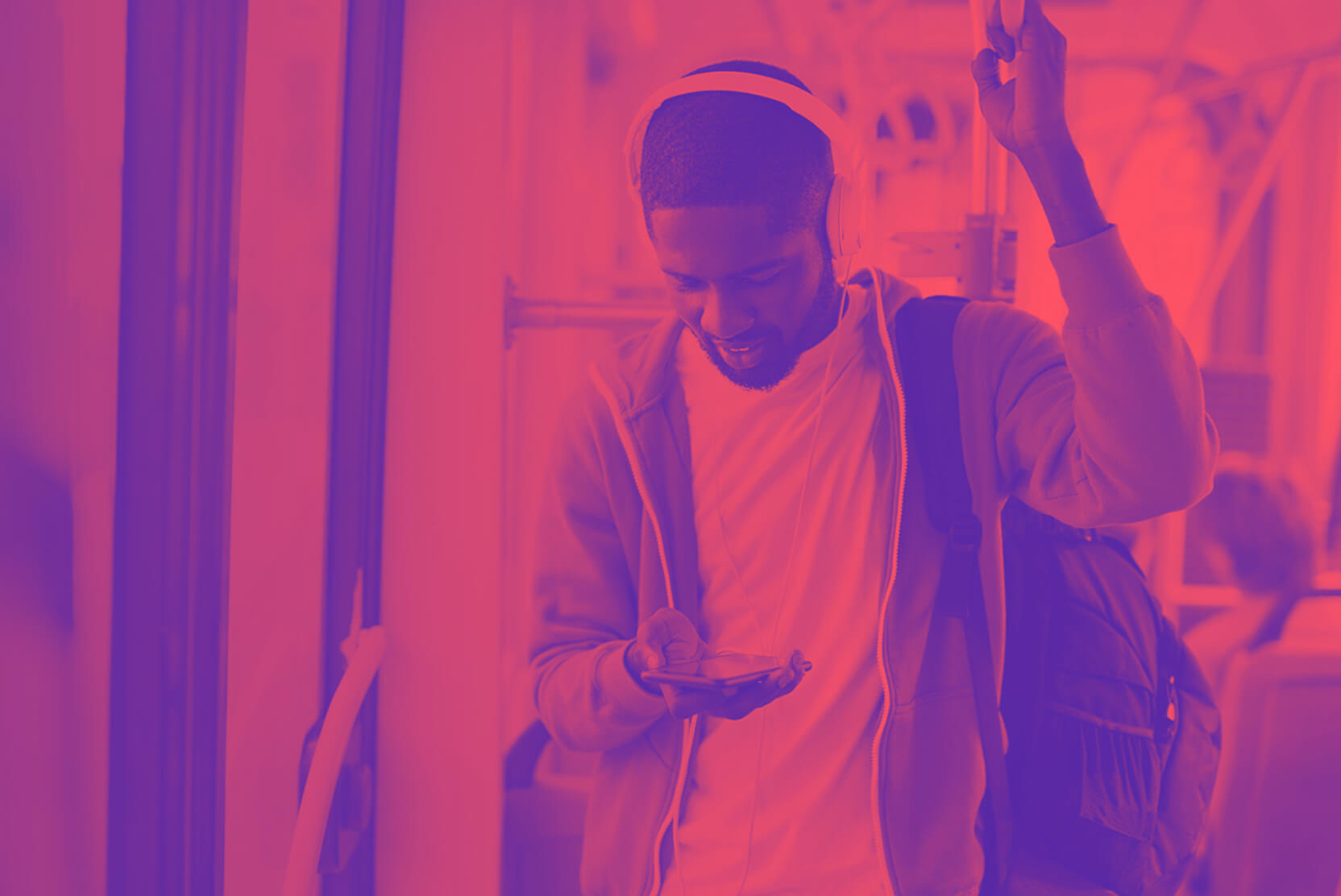
Newsletter
We care about protecting your data. Here’s our Privacy Policy.
Related news
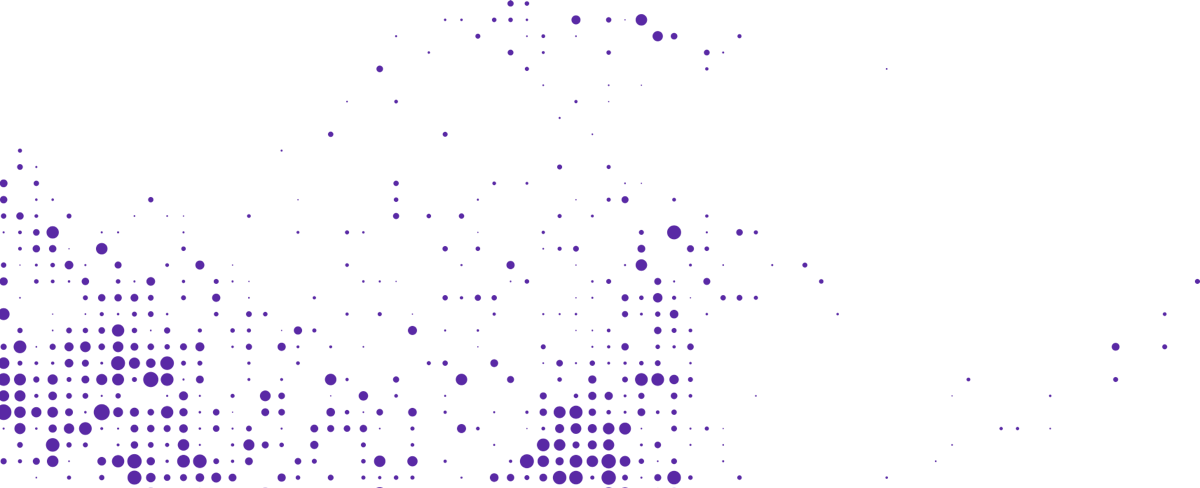
Start your journey with Passenger
If you want to learn more, request a demo or talk to someone who can help you take the next step forwards, just drop us a line.